1×1 Convolution based on the RISC-V Vector Extension
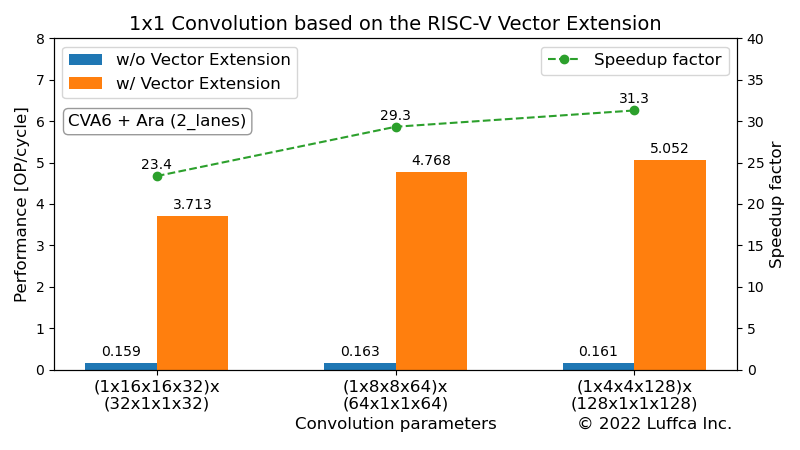
We have created a 1×1 convolution kernel based on the RISC-V Vector Extension (RVV) and evaluated its performance using an RTL simulator.
Click here for related articles.
- Running Auto-Vectorized Program on RISC-V Vector RTL Simulator
- Matrix Multiplication based on the RISC-V Vector Extension
- 1×1 Convolution based on the RISC-V Vector Extension (this article)
1×1 Convolution
A 1×1 convolution computes the dot product of each (h, w) input of size HxWxC with a filter of size 1x1xC for each output channel.
The pre-vectorized code with reference to TensorFlow Lite for Microcontrollers looks like this:
// output_data = 1x1_conv((input_data + input_offset), filter_data) with // input_data = [B, H, W, C], filter_data = [OC, 1, 1, C], // output_data = [B, H, W, OC] void OneByOneConvInt8(const int8_t* input_data, const int8_t* filter_data, int32_t* output_data, const int32_t input_offset, ...) { ... for (int batch = 0; batch < batches; ++batch) { for (int out_y = 0; out_y < output_height; ++out_y) { const int in_y = out_y; for (int out_x = 0; out_x < output_width; ++out_x) { const int in_x = out_x; for (int out_channel = 0; out_channel < output_depth; ++out_channel) { int32_t acc = 0; for (int in_channel = 0; in_channel < input_depth; ++in_channel) { int32_t input_val = input_data[Offset(input_shape, batch, in_y, in_x, in_channel)]; int32_t filter_val = filter_data[Offset(filter_shape, out_channel, 0, 0, in_channel)]; acc += (input_val + input_offset) * filter_val; } output_data[Offset(output_shape, batch, out_y, out_x, out_channel)] = acc; } } } } }
Note that input_data and filter_data are int8_t
type arrays, and output_data is int32_t
type arrays.
Ara
Ara is an implementation of the RISC-V Vector Extension developed by the Parallel Ultra Low Power (PULP) project. For an overview of Ara, see the related article Matrix Multiplication based on the RISC-V Vector Extension.
1x1 Convolution on Ara RTL Simulator
Ara can create an RTL simulator for Ara system combining CVA6 and Ara, using Verilator. This time, we used the minimal configuration 2_lanes.mk
with two 64-bit vector units.
Below is the console output when running the 1x1 convolution kernel.
$ cd $ARA/hardware $ app=1x1_conv_int8 make simv ... =================== = 1X1 CONV INT8 = =================== -------------------------------------------------------------------- Calculating a (1 x 16 x 16 x 32) x (32 x 1 x 1 x 32) convolution... -------------------------------------------------------------------- Initializing data... Calculating 1x1 conv without vector extension... The execution took 3300106 cycles. The performance is 0.159 OP/cycle. Calculating 1x1 conv with vector extension... The execution took 141214 cycles. The performance is 3.713 OP/cycle. Verifying result... Passed. -------------------------------------------------------------------- Calculating a (1 x 8 x 8 x 64) x (64 x 1 x 1 x 64) convolution... -------------------------------------------------------------------- Initializing data... Calculating 1x1 conv without vector extension... The execution took 3224180 cycles. The performance is 0.163 OP/cycle. Calculating 1x1 conv with vector extension... The execution took 109951 cycles. The performance is 4.768 OP/cycle. Verifying result... Passed. -------------------------------------------------------------------- Calculating a (1 x 4 x 4 x 128) x (128 x 1 x 1 x 128) convolution... -------------------------------------------------------------------- Initializing data... Calculating 1x1 conv without vector extension... The execution took 3247290 cycles. The performance is 0.161 OP/cycle. Calculating 1x1 conv with vector extension... The execution took 103775 cycles. The performance is 5.052 OP/cycle. Verifying result... Passed.
For 32, 64 and 128 input/output channels, we have achieved a speedup of 23-31 times over CVA6.
Summary
We created a 1x1 convolution kernel based on the RISC-V Vector Extension and evaluated its performance using Ara's RTL simulator.